Machine learning in healthcare, the future of medicine
"Do you trust your doctor?"
This is the somewhat puzzling question that Marzyeh Ghassemi, a Canadian medicine researcher will ask at the TEDxUofTSalon.
She then discusses the use of machine learning in healthcare and medical research.

This question is not asked to denounce bad practices or to replace health professionals with machines.
Nevertheless, it exposes several problems encountered today in the management and diagnosis of patients based on the data collected.
How to ensure, for example, that the doctor asks the right questions?
And as such, does he/she always get the right answers to determine the best treatment?
This is where HealthTech companies come in, as they seek to develop a type of machine learning* in healthcare that can optimize the collection and use of medical data.
*Machine learning? Detailed below, this is a category of artificial intelligence (AI) that can efficiently collect data and then develop new functionalities useful to healthcare professionals.
So how can machine learning assist healthcare professionals and optimize care and treatment? And how can it advance medical research?

Machine learning in healthcare, a solution to multiple problems
1/ To complete the use of EHR
Today, in the context of patient care, doctors and other health professionals will use the EHR (Electronic Health Record) and business software.
In France, the DPI for example, was introduced in 2021 and can be found in other countries under various names such as the EHR (Electronic Health Record) in the US.
Overall, the objectives are the same:
- Improve the quality, safety and efficiency of care,
- Engage patients and their families,
- Modernize the various public health systems,
- Ensure the privacy and security of personal information.
Unfortunately, the reality is quite different.
In fact, these projects are only effective in infrastructures where operations are more or less normal. This is not the case today for all institutions that do not have the same funds.
How can we ensure that the software is constantly updated in a context where priorities are turned towards other fundamentals (e.g., building renovation, restocking, etc.)?
The major problem of EHRs and medical software comes from this continuous need for updating. And for that, you need to call upon specialized engineers or developers. In other words, it costs money.
But without a continuous exploitation of these EHR, the collection of medical data is doomed to failure (80% each year on the number of data collected are lost).
Machine learning in healthcare could be, definitively, the solution for processing this medical data.

2/ And simplify the collection and use of medical data
At the time of the first examination
As we have seen, medical data can be collected via the EHR. However, without any optimization, erroneous data and/or data that are not necessarily useful for research can be obtained.
Note: In a 3-month survey by Kaiser Health News and Fortune Magazine, it states that 21% of US electronic medical records contain errors. Of course, this figure must be taken with perspective. Medical errors come from different sources.
But the data is also, and above all, obtained through the care givers, which is in constant contact with patients. Hence the need to provide them with professional tools that are efficient and adapted to their work pace*.
A study by the Healthcare Information and Management Systems Society revealed that out of a panel of 400 doctors and nurses selected in 11 different countries, 98% had already experienced a professional feeling of exhaustion similar to burn-out. In this type of situation, we know that fatigue necessarily affects the quality of work and the time spent with patients.

And during the diagnosis
After the fact, data collection tends to be used by doctors to draw conclusions about the situation of their patients, in order to make a diagnosis.
For this, they can use medical software. Nevertheless, these software do not necessarily ask the right questions. At least, they are quite limited, especially with all the data collected.
Take a patient who goes to a hospital and presents a particular disorder. The first reflex will be to ask the patient his medical file, his history...etc. In a second step, some tests will be performed and then a diagnosis will have to be made.
In this case, the doctor will go to the database already available. Then, he will make comparisons with already registered cases with similar symptoms. This is called the comparative method.
Besides the fact that this is time consuming, there is no guarantee that the patient will receive personalized treatment*.
*Note: an OHDSI study showed that if you took the medical records of 250 million patients in 4 different countries, only a tiny fraction would follow the same treatment for diabetes (10%), depression (11%) and hypertension (24%).
Each individual is unique and does not react in the same way.
Machine learning would therefore lay the foundations for personalized medicine.
Buy your first crypto easily with Galeon
Machine learning in healthcare: how does it work?
So, how could technicaly, machine learning be more efficient than all the tools used by doctors until now? How does it work?
Machine learning in healthcare is a "subcategory" of artificial intelligence (AI).
It allows the collection of data, the recording of questions asked by clinicians, all in an automatic way.
Once the process is well established and the machine has been fed with useful information, it can suggest new questions to guide healthcare professionals.
The advantage is that all this is done in record time. This is not the case with a human brain or a computer that processes tons and tons of information.
Beyond this aspect, machine learning could allow us to personalize the management of each patient without confusing him in a mass of health data that seem similar.
In other words, we stop considering that all patients with the same symptoms must follow the same treatment.
Ultimately, doctors would then have better tools to make decisions, saving time on their consultations, and regaining proximity with their patients.
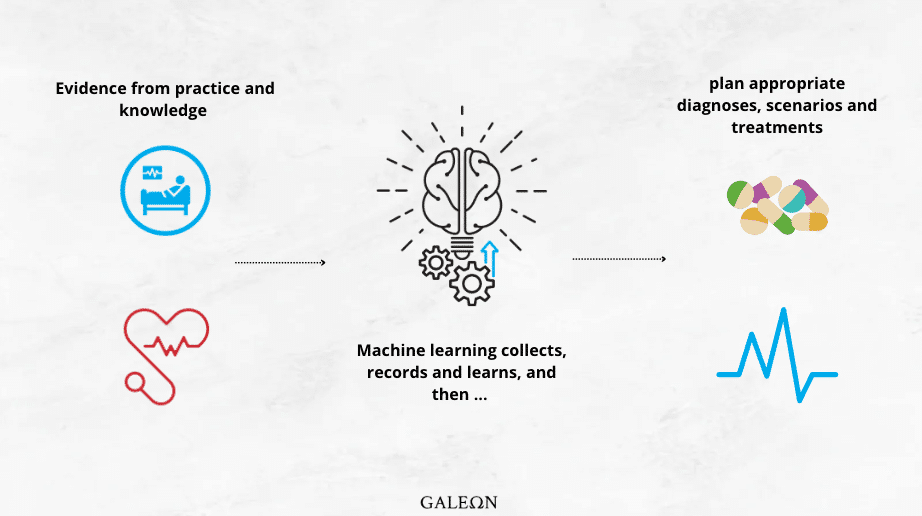
Other examples of machine learning and AI in healthcare
Other possible use cases for machine learning in healthcare
Atomwise is an American company developing an AI capable of comparing 10 to 20 million genetic codes per day in order to find the origin of diseases like Ebola or multiple sclerosis. Note that this is 100 times faster than pharmaceutical companies.
Caption Health combines AI and ultrasound technology to detect heart disease earlier. AI then allows to guide the ultrasound during the procedure, bringing a better image quality for a posteriori interpretation.
Freenome is used to provide images and other medical tests to detect stage 1 cancers.
Vicarious Surgical combines AI and robotic tools to assist surgeons in minimalist procedures. Thus, professionals can intervene remotely, without having to perform overly intrusive operations. In this field, we can also mention Intuitive Surgical.
In short, many machine learning and AI technologies are being developed, such as the evaluation of data and the establishment of accurate diagnoses, in order to improve the healthcare system.
Like Galeon, the idea is to personalize each patient experience, based on structured medical data.
Read also our article on blockchain and health.

The purpose of this article is above all to simplify technical concepts related to Web3, in order to make them accessible to all. These words only commit their author. It is not intended to advise the reader on his investments.
Would you like to be informed of Galeon's progress in advance?
Subscribe to our newsletter!